Streetwise
What is Streetwise about?
Spaces have positive or negative influences on us - they never behave neutrally. The "Streetwise" cooperation project of the Zurich Metropolitan Conference uses new approaches. It examines the everyday evaluation of places by the population and makes it visible.
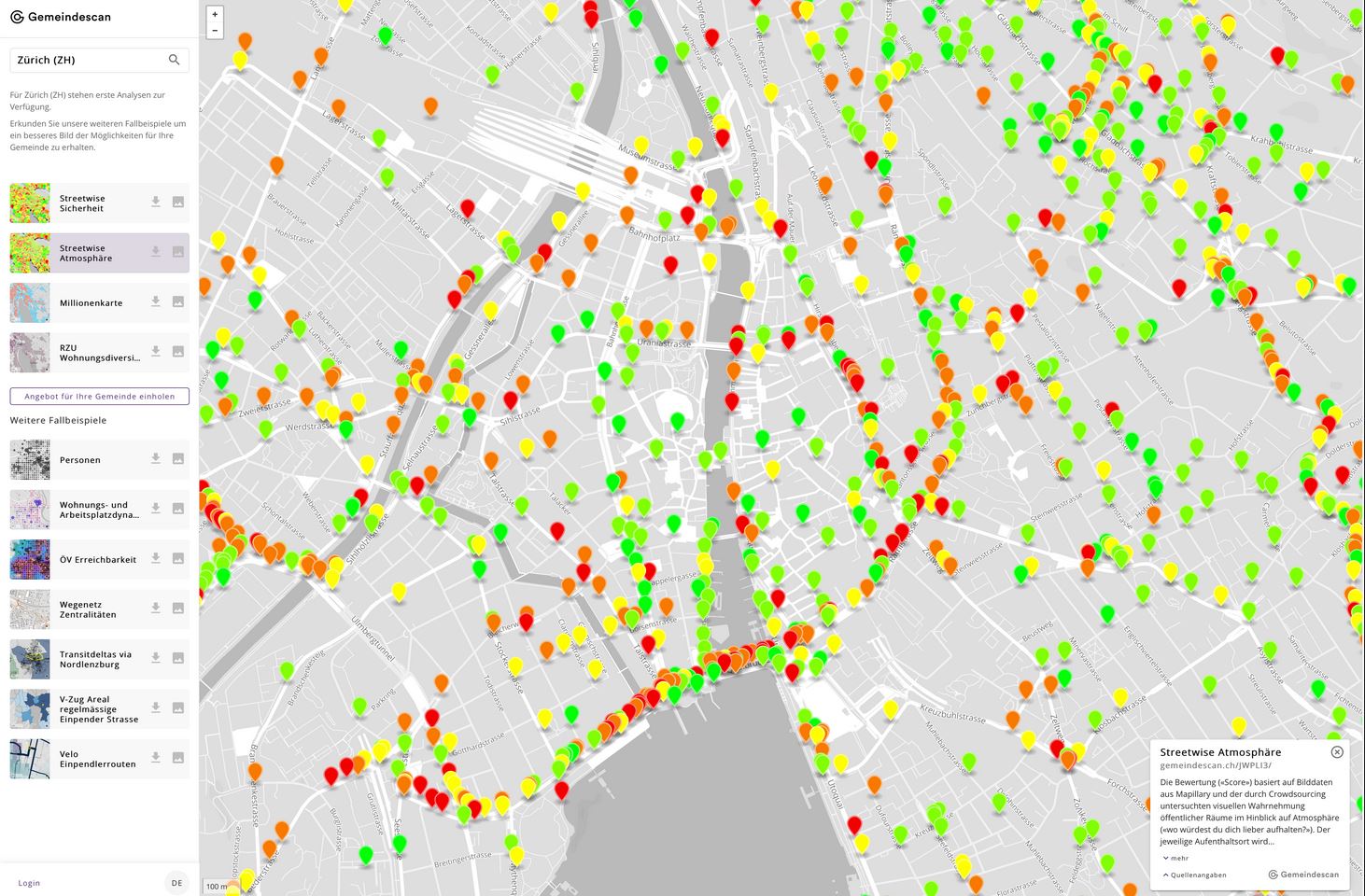
Streetwise records the human perception of spatial situations and uses the method of crowdsourcing to do so: a large number of people are shown pairs of images of public spaces online. By clicking on a picture, they each give a rating which is used to train an algorithm. Through a very large number of clicks the system learns to evaluate images independently. In this way, any spatial situation can eventually be evaluated automatically. The result is the first map of spatial quality in Switzerland.
There are no neutral spaces: either they help or they harm us. This is how clearly the architecture critic and author Sarah Williams Goldhagen sums up the importance of spatial qualities for our coexistence, our well-being or for the perception of security. The broken windows theory teaches us that there is a direct connection between the atmosphere - measured, for example, by the structural condition of urban areas - and crime. And Kevin Lynch proves in his epoch-making book "The Image of the City" that there are collective images of the city, that there is a public or general "image" of places and that we can capture this.
The connections between spatial perception and behaviour are central to the success of politically decided inward settlement development (->acceptance of redensification) or to safety (->intervention and prevention) - but also to every other policy and administrative domain that has a spatial component. This includes areas such as health, ageing as well as the planning of open space.
In the past we were confronted with a resource problem. The task was expensive, complicated, time-consuming and location-bound. At the same time, only selective data on the population's perception of spaces is available. The reason for this is that the collection of corresponding information has so far been associated with disproportionately large efforts in the form of surveys on specific topics and specific locations. The consequence of this is that in settlement development, for example, questions of spatial quality have been hastily dismissed as a "matter of taste" and the population's important perception of place has hardly been taken into account. The losses in efficiency and prosperity are difficult to estimate but they are likely to be considerable.
Today it is different. For some years now, machine learning has made it possible to solve complex problems such as the film tastes of millions of Netflix users or the consumption preferences of Amazon customers - or even to investigate spatial perception quantitatively. The point here is: While it is very difficult to define "safety" or "atmosphere" in space in a general and abstract way, it is very easy for people to judge a specific and concrete situation in terms of their feeling of safety or quality of stay, or to judge two situations at a time.
Streetwise takes advantage of this by having algorithms learn to assess spatial qualities by comparing many pairs of images. The aim of Streetwise is to make street wisdom, i.e. the knowledge and concern of the population, systematically usable for decisions by politicians, administration and civil society.
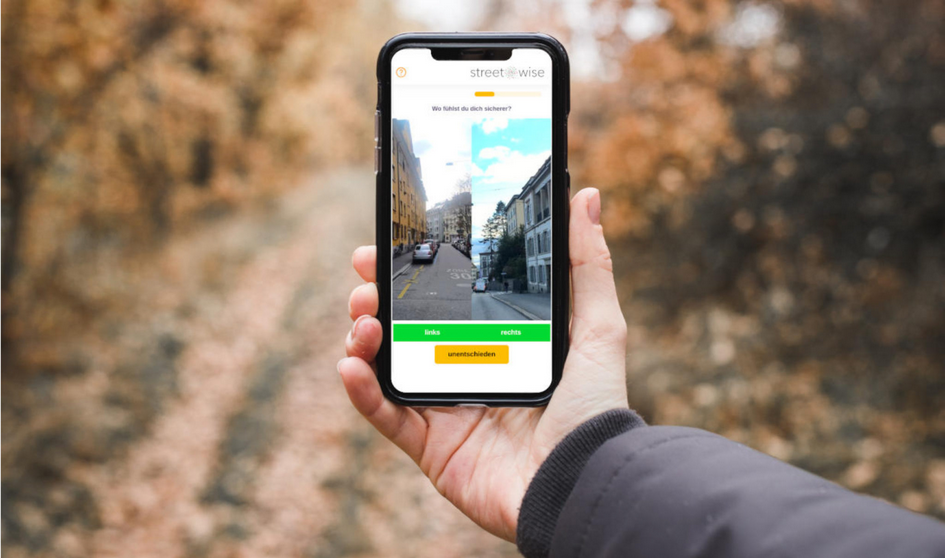
Further information
externe Seitehttps://streetwise.space/call_made